Artificial intelligence (AI) and machine learning (ML) are rapidly advancing fields that are continuously pushing the boundaries of technology and innovation. These areas have become central to progress in various sectors, from enhancing everyday tasks to solving complex problems in industries such as healthcare, finance, and autonomous driving. The advancement of AI and ML represents the remarkable achievements of human intellect and the vast potential of what the future may hold. But is everything as bright as it seems?
✅ AI Essay Writer ✅ AI Detector ✅ Plagchecker ✅ Paraphraser
✅ Summarizer ✅ Citation Generator
Key Takeaways:
- AI and ML are increasingly geared towards commercial applications, overshadowing basic research like AGI.
- Professionals in AI often face a stark contrast between their theoretical knowledge and the practical challenges of real-world software development.
- Much of what is considered innovative in ML is actually an application of established concepts, countering the notion of continuous breakthroughs.
- AI and ML professionals contend with a variety of challenges, including academic competition, research quality issues, communication barriers, business alignment, and ethical considerations.
Apparently not. Beneath the surface of these celebrated successes lie less-discussed challenges and downsides. These are not limited to technical issues but extend to ethical, professional, and practical aspects of working in AI and ML. Despite the growing enthusiasm and appeal of careers in these areas, the actual experience can significantly differ from public perception.
Without having a more comprehensive understanding of certain lesser-known aspects of a career in AI and ML, it isn’t easy to make decisions and plan steps. By drawing on real-life experiences and perspectives shared by professionals on Quora, we aim to present a balanced view crucial for anyone considering a career in these dynamic fields.
The Problem of Research Focus and Funding
In recent times, the course of AI and ML has been heavily influenced by their commercial applications. This focus, particularly on areas like targeted advertising and incremental advancements, has cast a shadow on more foundational research efforts, such as the pursuit of Artificial General Intelligence (AGI). This shift in focus raises concerns about the long-term implications and the direction in which these fields are heading.
A professional from the field, reflecting on their experience, shared on Quora, highlighting a significant change from exploratory research to commercially viable projects, where the primary goal often revolves around immediate market needs and profitability.
“The current trends in ML mean that no one wants to fund the basic research that might lead to AGI. Everyone just wants to make a deep learning system that makes you more likely to click on an ad.”
The impact of this shift is not just theoretical but has real-world consequences for those in the field. The same respondent, who has worked at Google Research, expressed their disillusionment: “Almost every published paper has to claim ‘State of the Art’… but this impedes real progress in the field.” They further elaborated on their decision to leave Google Research due to the company’s focus on consumer products and lack of interest in AGI.
This experience spotlights a broader challenge in AI and ML: securing funding for basic research. As the respondent observed, “It’s clear that mainstream companies and academia alike are not willing to fund basic research in this field, which is a real shame.” Such a funding environment limits the scope of research and potentially hampers groundbreaking advancements that could redefine our understanding and application of AI and ML.
Focusing on short-term, application-oriented research over long-term, foundational studies reveals a gap in the AI and ML community. It raises critical questions about the future of these fields and the balance between immediate commercial gain and the pursuit of more profound, potentially more transformative knowledge and capabilities.
The Hardships of AI Product Development
The first steps into AI product development are often taken with enthusiasm and high expectations. However, the reality of this path is filled with obstacles, particularly for those who come from a purely AI-focused background without extensive experience in software development. This gap between theoretical AI knowledge and the practicalities of software product development can lead to significant frustration.
One AI professional shared their insights on Quora, underscoring this point:
“It’s really, really hard to build AI-based software products.”
They elaborated on the unexpected difficulties faced in this process, especially for those without a strong foundation in software engineering.
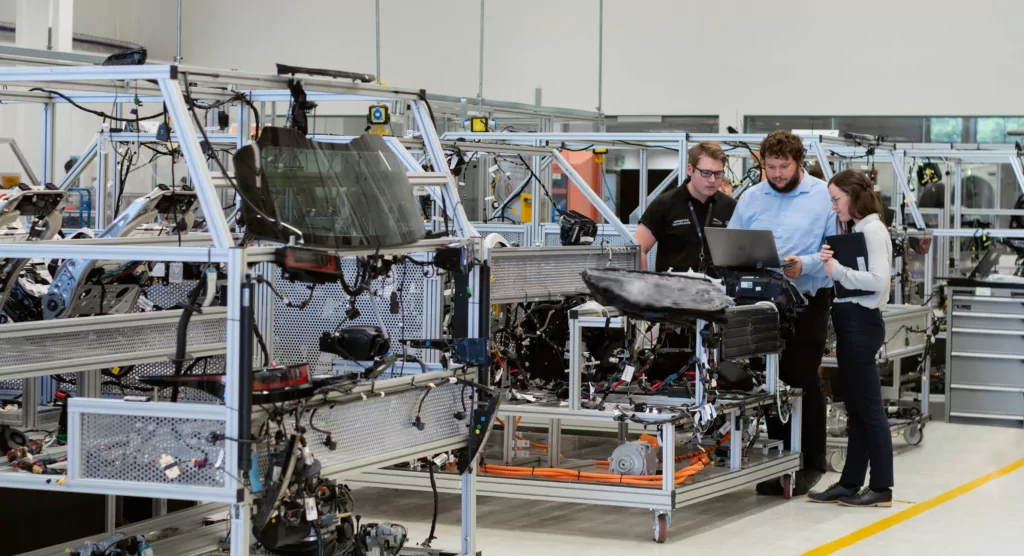
“You’ll likely discover to your disappointment that building real software products is a lot harder than building simple prototypes.”
Moreover, the field of AI and ML is characterized by its rapid pace of change, which can be both thrilling and disorienting. The same respondent said, “You may believe that all this great stuff you are learning… will be useful for a long time. To your dismay, you’ll find that every two years or so the field will have moved away from that.”
This fast-evolving nature of AI technology often leaves professionals struggling to keep up, bridging the gap between their current knowledge and the latest advancements.
The transition from theoretical learning to practical application in AI thus presents a unique set of challenges. It requires not just technical understanding but also adaptability to the ever-changing landscape of AI technologies. For many professionals, this journey brings a reality check, tempering their initial enthusiasm with a more grounded understanding of what AI development truly is.
The Misconceptions and Realities of Machine Learning
Machine Learning, often viewed through a lens of high-tech wizardry and revolutionary potential, harbors misconceptions that sharply contrast with its actual nature and capabilities. One prevalent misconception is the belief that ML work is continuously at the forefront of technological innovation. The respondent clears up this, stating,
“You peddle a largely technology hardware bounded product – that has not changed massively for about half 60–70 years or so.”
This means that much of what is celebrated as a novel in ML is, in reality, an evolution of long-standing technologies and principles. The user also addresses the over-simplification and glamorization of ML tasks. They note, “You largely get to be ‘innovative’ by simple circumventions of Physical attributal transformations.” This points to the fact that a significant portion of ML work involves adapting existing methodologies to new data or slightly different problems rather than groundbreaking discoveries.
Another critical aspect brought to light is the misalignment between public perception and the actual scope of ML. The professional comments, reflecting a reality where the foundational and often more challenging aspects of ML have been established in academic settings, while current industry applications might not always push these boundaries significantly:
“They call it Machine Learning like it’s hard and exciting… But all the hard lifting has been done by people locked behind Academia.”
Furthermore, the respondent highlights the industry’s tendency to rebrand old concepts as new breakthroughs, contributing to a cycle of hype. They mention, “You get to retread old ground – call it new.”
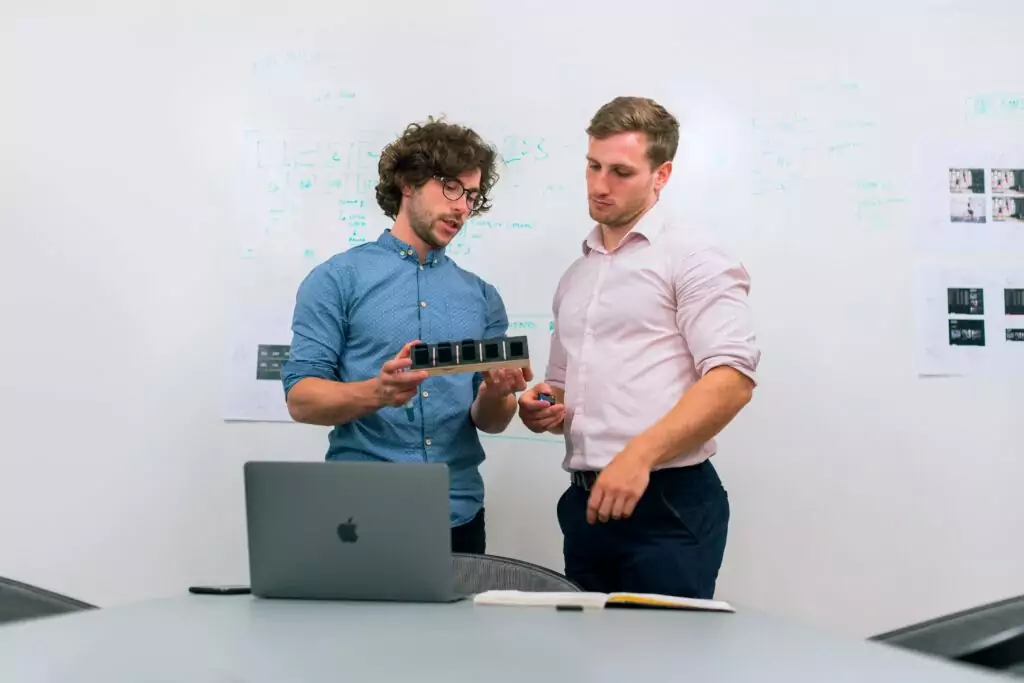
The response also touches on the broader community’s misunderstanding of ML. The professional points out, “Most of it is talk… People talk about ‘AI’ – half the time they’re unaware of what ML even is.” The gap in understanding, where the terms ‘AI’ and ‘ML’ are used interchangeably and often inaccurately exists, leading to a skewed perception of the field’s capabilities and limitations.
In summary, this subsection brings to light the gap between the common misconceptions about ML and its realities. It underscores the need for a more nuanced understanding of ML work, recognizing that much of what is considered innovative is an application or extension of existing theories and practices. This realization is crucial for those considering a career in ML, as it sets more realistic expectations about the nature of the work and the challenges involved.
Other Challenges in AI and Machine Learning
Beyond the core issues discussed in the earlier subsections, there are other challenges in AI and Machine Learning that, while perhaps less prominent, still significantly influence professionals in the field. These range from practical difficulties in daily work to broader concerns about the industry’s direction. To provide a comprehensive overview, these additional challenges are summarized in the following table:
> Category | > Description |
Competition in Academia | The intense competition for entry into graduate programs and subsequent job opportunities in AI. |
Review Quality in Research | The difficulty in maintaining high-quality peer reviews due to the sheer volume of submissions in AI conferences and journals. |
Volume of Research Papers | The overwhelming number of research papers published, making it hard to stay updated with new findings. |
Communication Gaps | Challenges in communicating complex AI/ML concepts to non-specialists, including software engineers and project managers. |
Business Understanding | The struggle to align AI/ML capabilities with business needs and decision-makers’ expectations. |
Data Quality Issues | The frequent encounter with poor quality or insufficient data is crucial for effective ML model training and implementation. |
Startup Challenges | The unique difficulties faced in AI startups, from unfounded technological promises to misaligned project goals and expectations. |
Ethical Considerations | The need to address ethical issues in AI applications and research, especially concerning privacy, bias, and societal impact. |
Follow us on Reddit for more insights and updates.
Comments (0)
Welcome to A*Help comments!
We’re all about debate and discussion at A*Help.
We value the diverse opinions of users, so you may find points of view that you don’t agree with. And that’s cool. However, there are certain things we’re not OK with: attempts to manipulate our data in any way, for example, or the posting of discriminative, offensive, hateful, or disparaging material.