Professor James Zou and his Stanford University team have questioned these AI detectors’ objectivity.
✅ AI Essay Writer ✅ AI Detector ✅ Plagchecker ✅ Paraphraser
✅ Summarizer ✅ Citation Generator
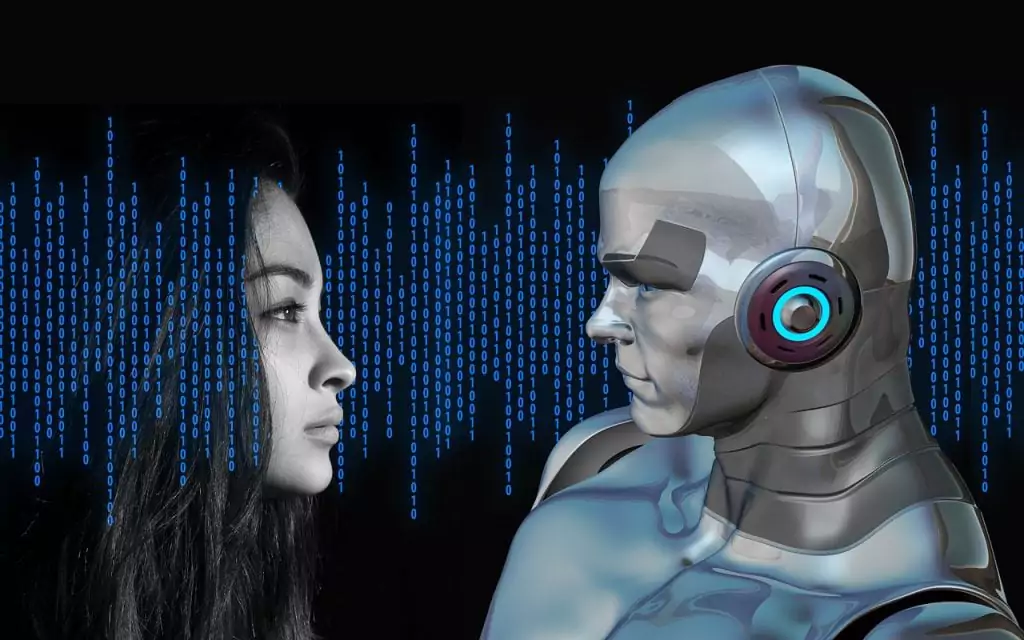
Key Takeaways
- AI detectors designed to identify AI-generated content have shown a bias against non-native English writers.
- The AI detectors are deemed unreliable and have been found to be easily manipulated.
- The AI detectors classified over half of TOEFL essays written by non-native English speakers as AI-generated.
As Andrew Myers from Stanford University reported, Artificial Intelligence (AI) detectors, which are designed to identify content generated by AI, have been found to be notably unreliable and display significant bias against non-native English speakers. This insight comes following a study conducted by scholars at Stanford University.
Unreliable Detectors
The AI detectors have emerged as a response to the high-profile launch of AI systems like ChatGPT. Developers and companies promote these detectors as tools to identify AI-created content, thus combating issues like plagiarism, misinformation, and cheating.
However, the Stanford study has revealed that these detectors are not as reliable as initially assumed. The unreliability is particularly evident when the actual author is not a native English speaker. The study showed that while the detectors were almost flawless in evaluating essays by US-born eighth-graders, they misclassified 61.22% of TOEFL essays (Test of English as a Foreign Language) written by non-native English students as AI-generated.
This discrepancy becomes more concerning as all seven AI detectors examined unanimously identified 18 out of 91 TOEFL student essays as AI-generated. Further, at least one of the detectors flagged a remarkable 97% of the TOEFL essays.
Bias and Ethical Concerns
“The detectors’ modus operandi raises questions about their objectivity,” states James Zou, a professor of biomedical data science at Stanford University and the study’s senior author. Zou explains that the detectors typically use a metric called ‘perplexity’, which correlates with the sophistication of the writing, where non-native English speakers naturally lag behind their US-born counterparts.
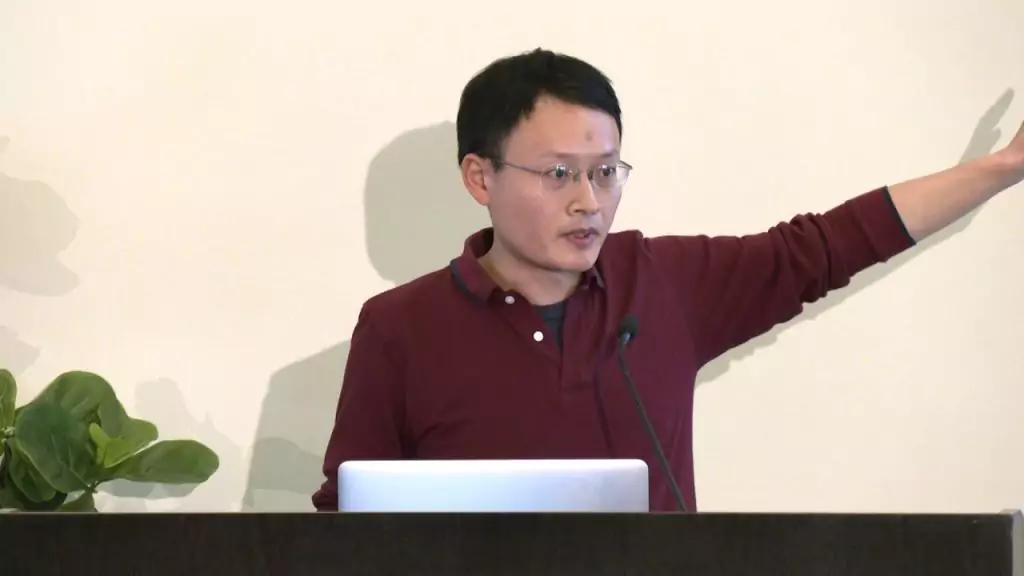
This reveals a bias that the Stanford team argues could lead to foreign-born students and workers being unfairly accused or penalized for cheating. Moreover, Zou points out that these detectors are easily circumvented through “prompt engineering,” making them an unreliable solution to the problem of AI-generated cheating.
Looking Forward
Zou offers some suggestions to address these issues. Firstly, reliance on these detectors in educational settings should be reduced, especially with high numbers of non-native English speakers. Secondly, developers should focus on more sophisticated techniques beyond perplexity or perhaps incorporate watermarks into the AI-generated content to indicate its origin. Lastly, efforts should be made to make the models less susceptible to circumvention.
With the current stakes for students, it is crucial that these technologies undergo rigorous evaluation and significant refinements. As Zou puts it, “The detectors are just too unreliable at this time, and the stakes are too high for the students, to put our faith in these technologies without rigorous evaluation and significant refinements.”
Related stories:
WolframAlpha: AI-Driven Knowledge at Your Fingertips
Codeamigo: The Emergence of AI-Powered Coding Education
Khan Academy’s AI Chatbot: A Transformation or a Challenge for Education?
Follow us on Reddit for more insights and updates.
Comments (0)
Welcome to A*Help comments!
We’re all about debate and discussion at A*Help.
We value the diverse opinions of users, so you may find points of view that you don’t agree with. And that’s cool. However, there are certain things we’re not OK with: attempts to manipulate our data in any way, for example, or the posting of discriminative, offensive, hateful, or disparaging material.